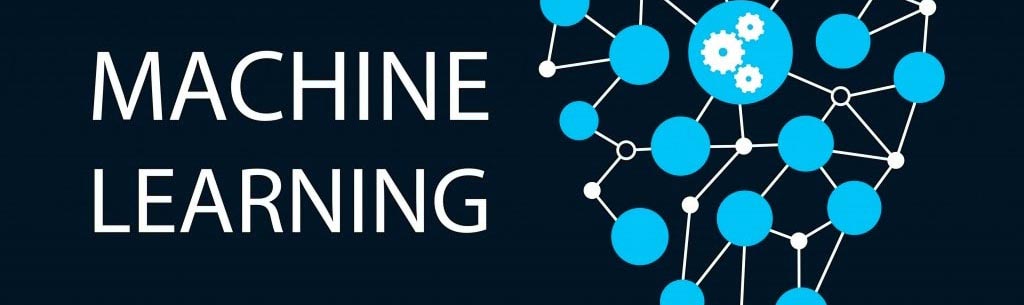
Introduction:
In today’s digital landscape, the rising sophistication of cyber threats has made cybersecurity a critical concern for businesses and individuals alike. Traditional security measures often fall short against advanced threats, leading to a growing interest in machine learning (ML) as a vital tool for enhancing cybersecurity. This blog post delves into the role of machine-learning in cybersecurity, its benefits, applications, and future trends.
What is Machine Learning?
Machine learning is a subset of artificial intelligence (AI) that enables systems to learn from data, identify patterns, and make decisions with minimal human intervention. By leveraging algorithms and statistical models, machine-learning systems can improve their performance over time as they are exposed to more data.
The Importance of Machine Learning in Cybersecurity
Cybersecurity threats are becoming increasingly sophisticated, making it difficult for traditional security solutions to keep pace. Machine learning offers several advantages that enhance cybersecurity:
- Threat Detection: ML algorithms can analyze vast amounts of data to identify anomalies and potential threats that would be impossible for human analysts to detect. By recognizing patterns associated with malicious activity, machine-learning systems can flag unusual behavior in real-time.
- Automated Response: In the event of a detected threat, machine learning systems can initiate automated responses, reducing the response time significantly. This proactive approach minimizes potential damage and helps organizations respond swiftly to incidents.
- Fraud Prevention: Financial institutions are leveraging machine learning to identify fraudulent transactions. By analyzing transaction data, ML algorithms can spot irregularities, helping organizations mitigate risks and protect their assets.
- Phishing Detection: Machine-learning can improve the identification of phishing attempts by analyzing email content, sender behavior, and metadata. This helps in preventing users from falling victim to such attacks.
Key Applications of Machine Learning in Cybersecurity
Machine-learning is transforming various aspects of cybersecurity. Here are some key applications:
1. Intrusion Detection Systems (IDS)
ML-powered IDS can analyze network traffic patterns to detect suspicious activities. By learning from historical data, these systems can identify potential intrusions and alert security teams before any damage occurs.
2. Endpoint Protection
Machine learning enhances endpoint protection by monitoring device behavior. It can detect anomalies such as unusual file access or unauthorized application usage, providing a robust defense against malware and ransomware attacks.
3. Security Information and Event Management (SIEM)
SIEM solutions incorporate machine-learning to correlate data from various sources, enabling organizations to detect complex threats and respond more effectively. By analyzing logs and events, ML algorithms can identify security incidents that may otherwise go unnoticed.
4. User and Entity Behavior Analytics (UEBA)
UEBA solutions use machine learning to establish baselines for user and entity behavior. By identifying deviations from these baselines, organizations can detect insider threats or compromised accounts, adding an additional layer of security.
Challenges in Implementing Machine Learning in Cybersecurity
Despite its advantages, integrating machine-learning into cybersecurity presents several challenges:
- Data Quality: The effectiveness of machine learning models depends on the quality and quantity of data used for training. Poor data quality can lead to inaccurate predictions and increased false positives.
- Adversarial Attacks: Cybercriminals are increasingly employing techniques to deceive machine learning models. Adversarial attacks can manipulate input data, making it crucial for organizations to continuously update and refine their models.
- Skill Gap: There is a shortage of skilled professionals who understand both machine-learning and cybersecurity. Organizations must invest in training and development to effectively implement ML solutions.
Future Trends in Machine Learning and Cybersecurity
The intersection of machine-learning and cybersecurity is an evolving field, with several trends shaping its future:
- Increased Automation: As machine learning technology advances, more cybersecurity processes will become automated, allowing organizations to respond to threats with greater speed and efficiency.
- Integration with AI: The convergence of machine learning with other AI technologies, such as natural language processing and deep learning, will enhance threat detection and response capabilities.
- Privacy-Preserving Machine-Learning: With growing concerns over data privacy, future ML solutions will focus on privacy-preserving techniques, allowing organizations to leverage data without compromising user privacy.
- Collaboration and Information Sharing: The future of cybersecurity will likely involve greater collaboration between organizations, allowing them to share threat intelligence and improve machine learning models collectively.
Conclusion
Machine learning is revolutionizing the cybersecurity landscape by providing innovative solutions to combat increasingly complex cyber threats. By leveraging its capabilities, organizations can enhance their security posture, improve threat detection, and respond more effectively to incidents. However, as the technology evolves, organizations must remain vigilant about the challenges and continuously adapt their strategies to stay ahead of cybercriminals. Embracing machine-learning in cybersecurity is not just a trend; it’s a necessity for safeguarding our digital future.
By optimizing for keywords like “machine learning in cybersecurity,” “cyber threats,” and “automated response,” this blog post aims to attract organic traffic from search engines while providing valuable insights into the role of machine-learning in protecting against cyber threats.
FAQs about Machine Learning in Cybersecurity
1. What is machine learning in cybersecurity?
Machine-learning in cybersecurity refers to the use of algorithms and statistical models to analyze data and identify patterns associated with security threats. It helps automate threat detection, response, and prevention, enhancing the overall security posture of organizations.
2. How does machine learning improve threat detection?
Machine learning improves threat detection by analyzing vast amounts of data to identify anomalies and unusual patterns that may indicate malicious activities. By learning from historical data, ML algorithms can recognize potential threats in real-time, allowing for faster response times.
3. What are some common applications of machine learning in cybersecurity?
Common applications of machine-learning in cybersecurity include:
- Intrusion Detection Systems (IDS)
- Endpoint Protection
- Security Information and Event Management (SIEM)
- User and Entity Behavior Analytics (UEBA)
- Fraud Detection
4. What are the benefits of using machine learning in cybersecurity?
The benefits of using machine-learning in cybersecurity include:
- Enhanced threat detection capabilities
- Faster response times through automation
- Improved accuracy in identifying fraudulent activities
- Proactive identification of phishing attempts and insider threats
5. What challenges are associated with implementing machine-learning in cybersecurity?
Challenges in implementing machine learning in cybersecurity include:
- Ensuring data quality for effective model training
- Protecting against adversarial attacks that can manipulate ML models
- Addressing the skills gap in the workforce regarding ML and cybersecurity knowledge
6. Can machine learning replace human security analysts?
While machine-learning can significantly enhance cybersecurity processes, it is not meant to replace human analysts. Instead, it acts as a tool to augment their capabilities, allowing them to focus on more complex tasks and decision-making rather than routine data analysis.
7. What future trends should we expect in machine learning and cybersecurity?
Future trends in machine-learning and cybersecurity may include:
- Increased automation of security processes
- Greater integration of AI technologies for improved threat detection
- Development of privacy-preserving machine learning techniques
- Enhanced collaboration and information sharing among organizations
8. How can organizations prepare to implement machine learning in their cybersecurity strategies?
Organizations can prepare by investing in training and development for their teams, ensuring high-quality data for model training, and regularly updating their machine-learning models to adapt to evolving cyber threats. Collaborating with cybersecurity experts and staying informed about the latest trends can also help.
9. What is the role of data quality in machine learning for cybersecurity?
Data quality is crucial for the effectiveness of machine-learning models. High-quality, relevant data ensures that models can accurately learn patterns and detect threats. Poor data quality can lead to inaccurate predictions and an increased number of false positives.
10. How does machine learning contribute to fraud prevention?
Machine-learning contributes to fraud prevention by analyzing transaction data and identifying irregularities that may indicate fraudulent activities. By continuously learning from past transactions, ML models can flag suspicious behavior in real-time, allowing organizations to take preventive action.
These FAQs can help clarify common questions and concerns readers may have regarding machine-learning in cybersecurity, further enhancing the value of your blog post.